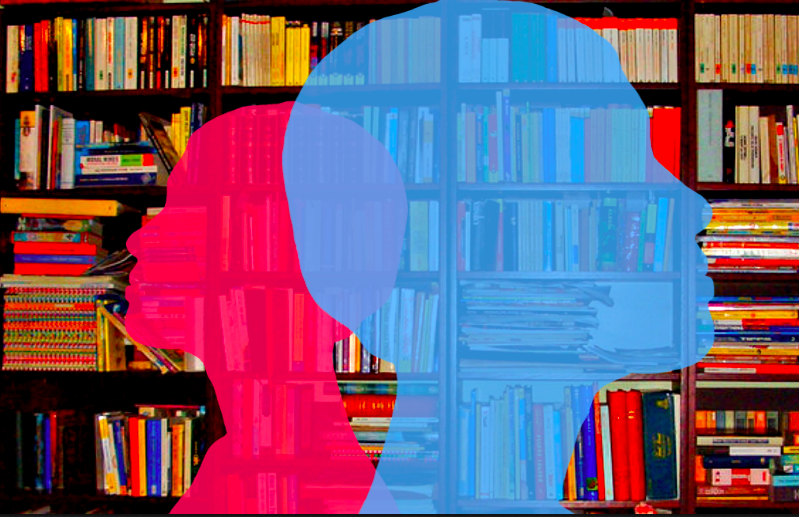
The technologies we are using to generate text—from auto-replies to articles—are learning the patterns in the set of texts we give them.
At the bottom of my Wikipedia page is a tag that identifies me as an “American women novelist.” If I were a man, the tag would read “American male novelist.” My gender should have nothing to do with my career, and yet there it sits, tied to my profession, as if the male novelists and I work in inherently different fields.
But one could easily make a cynical argument that we do.
Studies have shown women’s books are priced lower than men’s, women’s fiction is reviewed less often, and published less frequently in literary journals. Even books about women are less likely to win prizes than ones about men. The fields that men and women run through are different indeed: one of them has a lot more rough spots and potholes.
Over the past few months, as I’ve been looking at large text corpora, I often found myself thinking about gender inequality in the writing world. I wanted to collect banned books by men and women for a machine learning project (I planned to train two text-generating models on the different corpora and place them in conversation), but while banned texts by men are fairly easy to find in the public domain, banned texts by women proved much harder to come by.
As I searched for banned texts on Project Gutenberg, which hosts over 58,000 texts that can be downloaded free of charge, I began to wonder how many of the books—banned or not—were by women. One estimate came from Wikidata, where information found on Wikipedia pages—such as a person’s name, gender, or occupation—is stored in a way that’s machine readable. I found about twelve thousand people (writers, editors, illustrators, translators) who contributed to the corpus.
In this subset, men outnumber women by over 5 to 1. Although gender is not binary, I look at the number of men and women because this is the information available, or estimable, using name-based gender prediction tools.
I’d come to Project Gutenberg to find banned books for my bots, but I started to wonder what they would learn about writing if they were trained on this entire corpus. I have read a number of studies that identify patterns in language that are associated with one gender or another.
Researchers from Aalto and Helsinki Universities compared fiction by men and women in the British National Corpus and found that men use first-person plural (we, us) while women use second-person (you and your) more frequently. Men overuse certain nouns (e.g., ‘man’), women certain verbs (e.g., ‘thought’) and intensifiers (e.g., ‘much’ or ‘very’). The researchers note that such differences might be due to the gender of the intended audience, not the author, but this distinction quickly becomes murky.
What makes a book appropriate for one gender or another? When only the girls were invited to author Shannon Hale’s presentation—a teacher later told Hale, “the administration only gave permission to the middle-school girls to leave class for your assembly”, she noted:
“I talk about books and writing, reading, rejections and moving through them, how to come up with story ideas. But because I’m a woman, because some of my books have pictures of girls on the cover, because some of my books have ‘princess’ in the title, I’m stamped as ‘for girls only.’ However, the male writers who have boys on their covers speak to the entire school.”
If the language we use reflects what is expected of us—or if women’s books are only expected to be read by women—the fact that certain words are more commonly used by one gender or another strikes me as a symptom of systemic bias.
Like when I ran several of the essays I’ve written about technology through two different gender prediction systems and was identified as male by both. I suspect there is an imbalance in the training corpus and that I was called a man because the system had learned from the work it knew that men use words and phrases like “machine learning” and “biased data.”
What makes a book *appropriate* for one gender or another? Click To TweetI found over two million words of what I called “banned man” literature just by following the links from a single list of banned books. After poking around for a few hours, I collected around 800,000 words of banned woman literature from the public domain. I’d wanted at least a million words for each bot. I decided to revise my original machine learning plan and look at contemporary work instead.
I turned to Smashwords, where some books are sold and others may be freely downloaded, depending on the author’s wishes. On this site, the gender-related glut and shortages were opposite the ones I encountered on Project Gutenberg. I noticed far more women than men offering their one-hundred-thousand-word novels for free.
At this point, however, my interest in gender and language had eclipsed my interest in bot chatter. I was reading papers about statistical tests to determine which differences in word usage are significant and wondering things like how I could get my hands on a really big corpus. This is how I came across the Corpus of Contemporary American English (COCA): 560 million words from 220,225 texts collected between the years of 1990 and 2017.
I found this corpus dazzling, not just because I discovered my own work in it, but because when I opened the list of included writers and began to scroll through the names of fiction authors (who represent just a subset of the work), I was struck—in a positive way. Was the corpus as gender balanced as it appeared? I wrote to Professor Mark Davies of Brigham Young University, who maintains the corpus, and asked.
“Actually, the ‘balance’ just refers to the overall balance between the ‘macro-genres’ (spoken, fiction, etc) in COCA. As far as gender balance in fiction, I’ve never really designed the corpus to do that,” he said. He pointed me to the work of Doug Biber and Jesse Egbert, who have written about how to make a corpus representative—which is not a simple matter.
I appreciated Professor Davies’s candor, but was left with my question and a long list of fiction authors. I ran the first names through a gender predictor and the estimated ratio of women to men was fairly even. Men contribute more science fiction, women more of what is labelled as “juvenile work.” But I was frustrated by the uncertainty of the estimates.
The names are not always parsed correctly, the prediction just a guess, and I couldn’t see the women working under men’s names—people like George Madden Martin, Max du Veuzit, Lucas Malet, and Henry Handel Richardson, to name just a few. The irony that women, writing under men’s name to be heard, can so easily escape a search for female writers made me melancholy. I wanted to know who was in this corpus. I decided to try matching the names to biographical records in Wikidata again.
Using Wikidata via a tool called OpenRefine I could match just under half of the subset of five thousand names I tried. Not all of the names matched the correct person. For example, Elizabeth Evans—who is the author of six books and the recipient of an NEA fellowship—does not have a Wikipedia page, but she was matched to another person with the same name. As I was interested only in gender, I accepted this match—it seemed reasonably likely that the gender would be correct. Of the matched names, forty percent belonged to women.
I abandoned this line of inquiry, but I was left with my questions: Who is included in our corpora? Who is not? Whose voice am I hearing? What story does it tell? For the English Wikipedia, according to the estimates I’ve seen, over 80% of the contributors are male. The story there—our history—is disproportionately about men, and the biographies of men outnumber those of women significantly (the latest estimate I’ve seen shows just under 18% of the biographies are of women). I suspect the 40/60 imbalance in my COCA gender estimate belongs more to Wikipedia than COCA, but I know nothing more than that I observed it.
In the case of Project Gutenberg, the work is primarily by male authors and any patterns in the language that belong to men are magnified by this imbalance. If male authors use the word “man” more often than female authors do—as the researchers noted in their study of the British National Corpus—having five times more male than female authors gives that word an even greater prominence.
For the English Wikipedia, over 80% of the contributors are male. Click To TweetI think about how imbalances in our corpora magnify bias, not just in subject matter (stories about male characters or biographies of men), but in the words we see and choose. The technologies we are using to generate text—from auto-replies to articles—are learning the patterns in the set of texts we give them. And these technologies, in turn, are not only writing for all of us, but imposing the patterns they’ve learned. Not all people who write (or read) about technology are men, but the story the artificial intelligence knows, based on the words and the associations made from its training corpus, says otherwise.
I would love if my gender weren’t tied to my work, or diagnosed and misdiagnosed by technologies that reflect the biases I work against every day. I am a woman. I am a writer. The 1500 words I’ve written here won’t swing the gender balance in any large corpus, but I’m putting them out into the world, and I hope they will be counted.